The ocular proteome is a first collection of selected protein atomic structures targeted by genetic mutations in inherited eye disease. Ocular proteome collection of protein structures was created for the protein stability analysis for biochemical and next generation sequencing data on protein mutant variants, express analysis of effects of genetic mutations from the level of protein structure, and in silico analysis of genotype-to-phenotype relationships. Prediction of genetic disease phenotype change from the atomic level of protein structure was previously described (Sergeev et al, 2010, 2013). A global mutagenesis method used to generate the effect of genetic mutation at the protein structure was previously described (McCafferty C.L. & Sergeev Y.V., Sci. Reports, Sci. Data, 2016). All information to generate protein structures was obtained from public databases.
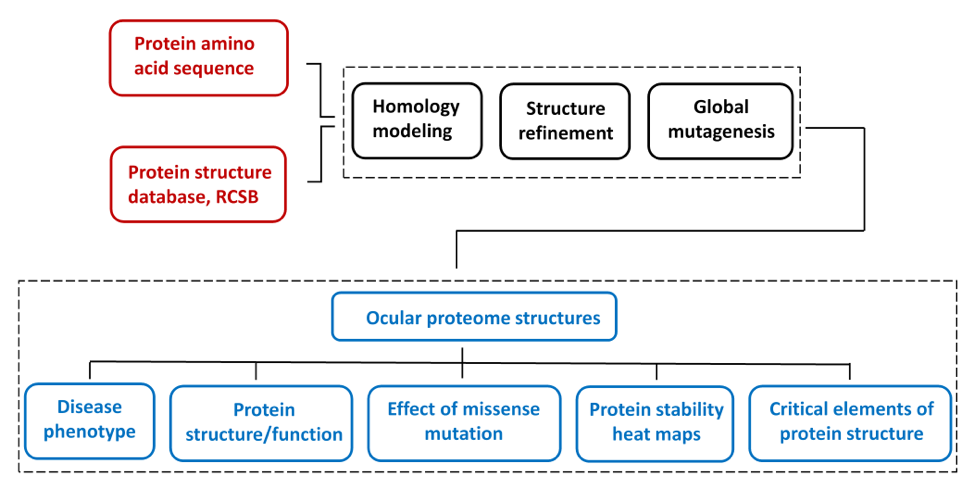
Human protein structures were generated by homology modeling using human protein amino acid sequences and the experimental atomic structures of proteins from other species as structural templates. This approach was employed for human proteins, which often does not have crystal structure available. In addition, some clinically important human proteins are represented by their protein structural domains if structural templates are not available for a whole protein..
The limited dataset of protein structures from inherited eye disease was selected from the network of nearly 1700 human disease-related proteins from Genetics Home Reference . For each modeled protein, the severity of genetic perturbations evaluated from the protein atomic structure. Briefly, the protein stability for each mutant variant was modeled in silico using the protein atomic structure perturbed by a missense mutation. A global landscape of protein stability changes was obtained by using a semi-empirical Gibbs free energies (FoldX) converted into unfolding propensities through the unfolding mutation screen program. A detailed description of the algorithm could be found in our publications (McCafferty C.L. & Sergeev Y.V., Sci. Reports, Sci. Data, 2016). A global mutation landscape generated for each protein to show possible outcomes of genetic changes at the protein atomic level for any possible missense mutation. Mutation landscapes are presented as heat maps and colored ribbon structures to show elements of protein critical for protein stability. Information about the homology model, heat maps, and critical residues associated with a severe change of protein stability is available from the database.
The accuracy of prediction of mutation effects was verified using protein atomic structures and thermodynamic data. For this purpose, the in silico unfolding propensities were extensively tested with 1391 mutant variants from 16 protein crystal structures from PDB (McCafferty C.L. & Sergeev Y.V., Sci. Reports, 2016). The unfolding propensities were compared with ones calculated from the experimental Gibbs free energy changes obtained from the ProTherm database. The analysis showed that the proteins from the validation dataset had an average of 77.9 ± 9.1% correct matches between the experimental and computed unfolding. The method was then applied to analyze the mutations and critical residues for different proteins from genetic disorders.
References
- McCafferty, C. L. and Sergeev, Y. V. (2016). In silico Mapping of Protein Unfolding Mutations for Inherited Disease. Scientific Reports. 6, 37298; doi: 10.1038/srep37298
- McCafferty, C. L. & Sergeev, Y. V. (2016). Dataset of eye disease-related proteins analyzed using the unfolding mutation screen. Scientific Data 3: 160112 doi: 10.1038/sdata.2016.112
- Sergeev Y.V., Vitale S., Sieving P.A., Vincent A., Robson A.G., Moore A.T., Webster A.R., Holder G.E. (2013). Molecular modeling suggests two classes of missense variants with mild and severe XLRS phenotypes, Human Molecular Genetics, 34(6):4756-4767.
- Sergeev Y.V., Caruso R., Meltzer M.R., Smaoui N., MacDonald I.M. & Sieving P.A. (2010). Molecular modeling of retinoschisin with functional analysis of pathogenic mutations from human X-linked retinoschisis. Human Molecular Genetics, 19(7):1302–1313.
Contributions
- Caitlyn McCafferty wrote a Python code for unfolding mutation screen.
- Francisca Wood Ortiz developed a Python code for the protein network mutation perturbation.
- Dr. Mikhail Laryukhin, Divyang Mago, and Samuel Hailai created the Proteome GUI interface.
- Dr. Yuri Sergeev supervised this project and performed molecular modeling.
Servers and programs used
- UNIPROT, http://www.uniprot.org
- RCSB, http://www.rcsb.org
- PROMALS3D, http://prodata.swmed.edu/promals3d/promals3d.php
- YASARA, http://www.yasara.org
- PHYRE2, http://www.sbg.bio.ic.ac.uk/phyre2/html/page.cgi?id=index
- RaptorX, http://raptorx.uchicago.edu/StructurePrediction/predict